Introduction
As digitalization takes the world by storm, systemic investment platforms find themselves grappling with vast amounts of complex data. Efficient data management is now more than just an option – it’s a critical necessity for informed decision-making and successful strategic planning. Knowledge Graphs, with their sophisticated mechanisms for data integration and understanding, provide an innovative solution to this challenge. This blog post delves into the compelling concept of leveraging Knowledge Graphs to construct a data lake, a vast repository of raw data, within systemic investment platforms.
Understanding Knowledge Graphs: More Than Just Data Linkage
Knowledge Graphs present a revolutionary way of storing and managing data by acknowledging and leveraging the intricate relationships between data points. They excel in unifying structured and semi-structured data, leading to a more comprehensive understanding of the information at hand.
Two essential components that make Knowledge Graphs distinctively powerful are semantic modeling techniques and ontologies. Semantic modeling breathes life into raw data by capturing domain-specific concepts and relationships, adding relevant context. Ontologies offer a structured framework that details the types, properties, and relationships between the entities within a specific domain. These components together equip Knowledge Graphs with an exceptional ability to integrate and analyze data.
Notably, Knowledge Graphs come with an inherent capability to infer new knowledge. They can intuitively recognize patterns and relationships, thus deducing additional insights that aren’t explicitly mentioned in the original data, a feature that sets them apart from traditional data management techniques.
The Power of Data Lakes in Systemic Investment Platforms
Data Lakes provides an effective solution to handle the volume and variety of data typical in systemic investment platforms. They offer centralized storage for raw data in its native format – structured, semi-structured, and unstructured – paving the way for advanced analytics and machine learning applications.
The Practical Magic of Knowledge Graphs for Data Lake Development
Knowledge Graphs play a pivotal role in the development of a data lake, particularly in three key areas:
- Data Integration: They enable the integration of data from diverse sources and formats into a unified, context-rich data lake. Efficient extraction, transformation, and loading of data become streamlined, resulting in a comprehensive data repository.
- Semantic Representation: The construction of a knowledge graph involves semantic modeling techniques and ontologies. This helps in capturing and representing domain-specific concepts, relationships, and contextual information within the data lake.
- Querying and Analysis: Knowledge Graphs facilitate the use of graph query languages and analytical tools to perform advanced data querying and analysis. This allows for complex traversals and explorations of data, leading to meaningful, actionable insights.
Reaping the Benefits: Knowledge Graphs in Systemic Investment Platforms
The integration of Knowledge Graphs into a data lake brings numerous benefits to systemic investment platforms. Efficient data integration and enriched semantic representation allow for improved data-driven decision-making, systemic risk assessment, portfolio optimization, and the identification of investment opportunities. The inferential capabilities of Knowledge Graphs also offer a more comprehensive understanding of the investment landscape, fostering better strategic decisions.
A Real-World Scenario: Knowledge Graphs in Action
Consider a systemic investment platform dealing with a broad range of investment products and interacting with multiple data sources, each with its own unique format. The organization decides to leverage Amazon Neptune, a fully-managed graph database service, to build a knowledge graph-based data lake.
They start by identifying entities (investors, investment products, market indicators, etc.) and relationships, translating them into a knowledge graph. Now, the organization can perform sophisticated queries that traverse these relationships, such as analyzing the impact of news events on specific stocks within certain sectors.
By integrating all the data in a single, queryable platform, the organization can derive comprehensive insights, fostering informed decision-making and unveiling novel investment opportunities that were previously hidden in the expansive web of unconnected data.
Beyond Amazon Neptune, several other graph databases like Neo4j, another popular graph database solution, are also being widely adopted for similar use cases. It facilitates efficient data modeling, querying, and reasoning over data relationships. Just like Amazon Neptune, Neo4j helps organizations unlock the potential of their data through knowledge graphs.
Recommendations for Implementing Knowledge Graphs
If you’re considering leveraging knowledge graphs for similar use cases in your organization, here are some key recommendations:
- Identify Your Entities and Relationships: This initial step is crucial for the successful construction of a knowledge graph. Thoroughly understanding your data’s entities and their relationships will guide the subsequent development process.
- Leverage Semantic Modeling: Semantic modeling techniques capture domain-specific concepts and relationships, adding crucial context to your data. This enables your knowledge graph to infer new knowledge and provide richer insights.
- Plan Your Data Integration Process: Consider the formats and structures of your different data sources. Planning how to extract, transform, and load this data into the knowledge graph is a critical stage in the process.
- Master Graph Query Languages: Knowledge graphs are only as good as your ability to query them. Mastering graph query languages like SPARQL or Cypher will maximize the insights you can derive from your data.
- Think Scalability: As your organization and data grow, so will your knowledge graph. Ensure that the graph database solution you select can scale to match your needs.
Conclusion
In the era of big data, Knowledge Graphs have emerged as an essential tool in data management for systemic investment platforms. Their powerful capabilities of data integration, semantic representation, and inferential knowledge provide unparalleled insights, driving data-driven decision-making, systemic risk assessment, and portfolio optimization.
Whether you choose to use Amazon Neptune, Neo4j, or any other graph database service, the integration of Knowledge Graphs and Data Lakes is set to revolutionize the landscape of systemic investment platforms. By harnessing the power of these sophisticated tools, organizations can unlock the full potential of their vast data assets, making well-informed, data-backed decisions that lead to more robust investment strategies and portfolios. The future of systemic investment is here, and it’s powered by Knowledge Graphs and Data Lakes.
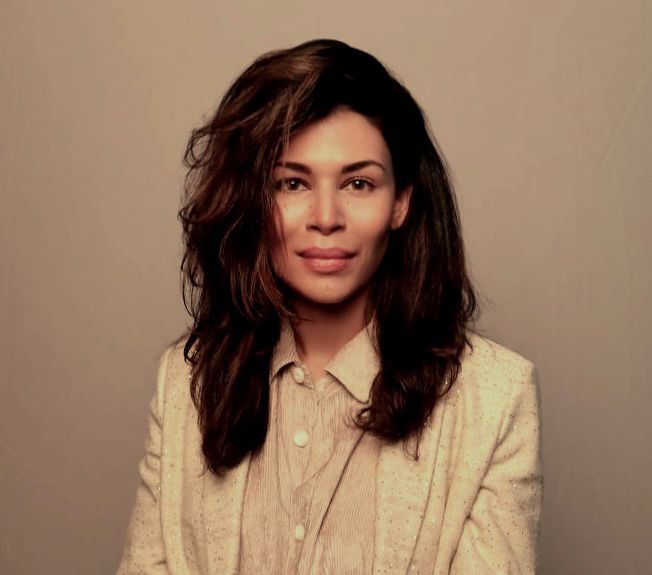
About The Author
I am Bhavna Chamoli, incredibly passionate about the potential of artificial intelligence (AI) and machine learning to revolutionize businesses. My focus is on exploring how AI can advance various industries and bring about transformative changes.
With a strong background in data analysis and machine learning, I continuously seek innovative ways to harness AI’s power for business enhancement. I firmly believe that AI can improve decision-making, optimize operations, and uncover valuable insights from complex datasets.
Bhavna Chamoli actively work on developing predictive models, automating workflows, and extracting insights from diverse data sources. By pushing the boundaries of AI research and application, I aim to discover new solutions that can propel businesses to greater success.
Bhavna Chamoli also dedicated to sharing knowledge and collaborating with others. Through workshops, presentations, and partnerships, I strive to spread awareness about advanced AI concepts and foster meaningful discussions within the business community.
If you’re interested in exploring how AI can benefit your business or simply want to learn more, feel free to connect with me on LinkedIn https://www.linkedin.com/in/bchamoli/ or visit my website, www.bhavanachamoli.com.
Let’s unlock the possibilities of AI together and pave the way for a future of remarkable advancements.!
Leave a Reply